Study adopts name-analysis to investigate bias in the Indian Judiciary
Inequalities across groups defined by gender, religion, and ethnicity are present in almost all societies and cultures. Therefore, the judicial system of a particular country may have unequal representation, mirroring the unequal access of different people to the legal profession.
Trending Photos
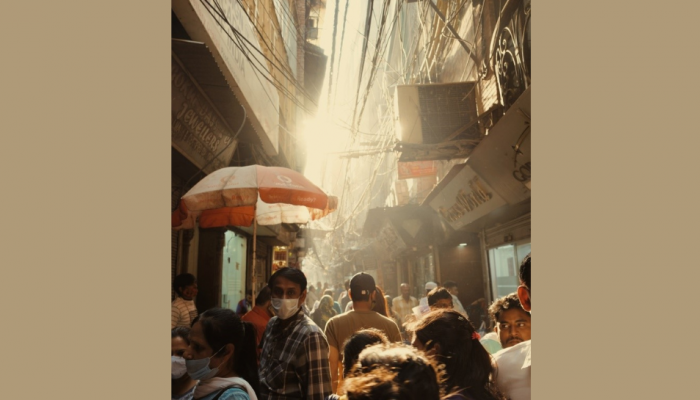)
A study from the Center for Global Development, ‘In-Group Bias in the Indian Judiciary’ (2023) examined bias in India's courts, investigating whether judges deliver more favourable treatment to defendants with similar backgrounds or identities. This issue has yet to be widely studied in the courts of lower-income countries. The research group focused on gender, religion, and caste in India's lower courts, examining whether unequal representation has a direct effect on the judicial outcomes of women, Muslims, and lower castes in an anonymised dataset of 5 million criminal court cases from 2010 to 2018.
Method of analysis
The eCourts platform does not provide demographic metadata on judges and defendants, but the research group was able to determine the characteristics of interest, namely gender and religion, from their names. In order to conduct the analysis, the research group trained a neural net classifier to assign gender and religion based on the text of names and apply it to our case dataset to assign identity characteristics to judges, defendants, and victims.
They use two databases of names with associated demographic labels to classify gender and religion (Muslim and non-Muslim). They then trained a neural net classifier to predict the associated identity label for pre-processed name strings using a bidirectional Long Short-Term Memory (LSTM) model.
The LSTM classifier was able to understand a text fragment within context, which improves accuracy over standard fuzzy string matching methods. For instance, the LSTM classifier can accurately identify the religious classification of a name based on the context of the word. The LSTM classifiers were trained for gender and religion using labelled databases, and the trained classifiers were applied to eCourts case records.
The judge and defendant names were then pre-processed and applied to the trained classifier to form a predicted probability for gender and religion. The results were 97% accurate.
Caste identity is one of the most important social distinctions in India, so it is vital to explore how bias impacts different groups. Unfortunately, caste is also very complex and hierarchical, making it difficult to specify binary in-groups and out-groups. Consequently, identifying caste based on names is quite the challenge, and the researchers were not able to develop a correspondence between names and specific castes. This is because, according to the researchers, individual names do not identify caste as precisely as they identify religious or gender identity; the caste significance of names can also vary across regions. Thus, the research group decided to define a caste identity match as a case where the defendant's last name would match the judge's last name, examining whether judges deliver more favourable outcomes to defendants who share their last name.
Results
The study found no gender-based or religion-based bias in criminal cases in India, but they found some in-group bias among social groups with shared uncommon last names. They also found no evidence of in-group bias among judges in terms of race/ethnicity, gender, or religion. This is in contrast with studies in other jurisdictions, where researchers have tended to find large effects.
For caste bias, even considering the difficulties of analysing it, the report found that a judge-defendant name match increases the likelihood of acquittal by 1.2-1.4 percentage points. This result suggests that there is caste-based in-group bias in groups made of individuals with less common names.
Namsor launches new software for classifying Indian names
This report shows the relevance of name analysis to analyse bias in situations where sensitive data such as gender, caste, and religion are not provided. While Namsor was not used in the academic study ‘In-Group Bias in the Indian Judiciary’ (2023), which involved training a custom AI model, Namsor used a similar approach and launched an AI model for Indian name classification by geography (state or union territory), by religion and by caste group. One benefit of the model is that it can be applied to any state or union territory of India, not just Delhi. Namsor could not assign names to specific castes but was able to train a model to recognise caste groups (Scheduled Castes, Scheduled Tribes, Other Backward Classes, General). One significant use case for the technology is to measure biases in other AI algorithms.
(Above mentioned article is a consumer connect initiative, This article is a paid publication and does not have journalistic/editorial involvement of IDPL, and IDPL claims no responsibility whatsoever.)
Live Tv