Exclusive: How Machine Learning And Self Driving Vehicles Are Making Instant Last-Mile Delivery A Reality
Real-time applications of machine learning and self-driving technologies are redefining the future of last mile delivery industry.
Trending Photos
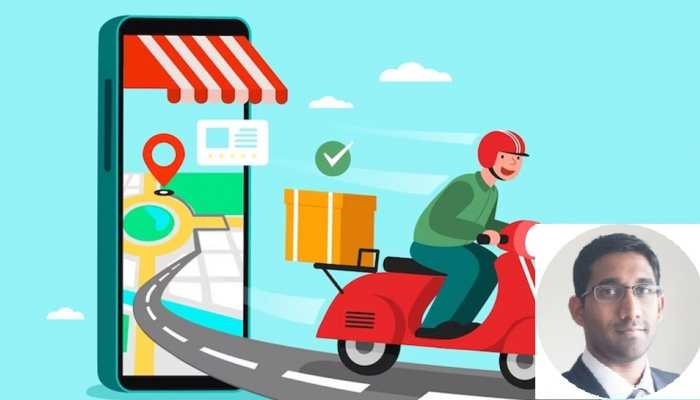)
Imagine having your groceries or favorite dish at your doorstep within 15 minutes of placing an order. Thanks to real-time machine learning and routing optimization algorithms, this is no longer a distant dream but a growing reality. To look deeper into the last-mile delivery, we talked to Dr. Aditya Gopi Dodda,a real-time machine learning expert and industry veteran in developing real-time applications using machine learning and optimization techniques. "The advancements in real-time machine learning have enabled a level of efficiency that was unthinkable a decade ago. Using algorithms that can make hundreds of thousands of calculations in a matter of seconds, we've been able to cut down delivery times dramatically," explained Dr Aditya Gopi Dodda.
The Boom of Last-Mile Deliveries
In the past decade, last-mile deliveries have experienced an unprecedented boom. "Companies like Swiggy and Uber Eats have harnessed algorithms that quickly match drivers with orders, considering real-time traffic data and driver availability. This optimization has led to a surge in same-day deliveries. At the heart of this transformation is the intricate and complex process of solving "assignment" problems-matching local drivers with local orders within seconds," said Dr. Dodda
Understanding the Complexity
The complexity in last-mile delivery stems from the need to solve a variant of the Vehicle Routing Problem (VRP) in real-time. As an order is placed, all the vehicles in the vicinity of the store and customer are identified. Then, for a densely populated area, the optimization module must consider a myriad of constraints like time windows, vehicle capacities, driver preferences, customer preferences, traffic conditions, road restrictions, fuel consumption, and even regulatory compliance. "We find ourselves dealing with the Multi-Depot VRP with Time Windows, where there are multiple starting locations for drivers and specific delivery time frames. These problems are in the category of Mixed Integer Linear Programming (MILP) which are solved using innovative algorithms that give close to optimal solutions quickly which allows us to navigate these multifaceted complexities in near real-time. Traditional methods would be far too slow for the dynamic and unpredictable nature of last-mile delivery," said Dr. Dodda.
The Need for Speed
In the world of last-mile delivery, time is of the essence. Traditional e-commerce platforms (Amazon, Flipkart) doing multi day shipping can afford to use more traditional (slower) optimization methods, but companies like Swiggy or UberEats must use real-time optimization techniques and machine learning. A delay of even 10 seconds in assignment might translate to unpleasant customer experiences, such as a delayed meal for a hungry customer.
"Typically, machine learning models are leveraged that continuously learn and adapt to a plethora of variables such as driver location, order priority, weather conditions, vehicle condition, historical delivery patterns, customer feedback, special handling requirements, and even localized events like concerts or sporting games that might affect traffic. These models can predict and preempt delays, orchestrating a symphony of deliveries that ensures every order reaches its destination as efficiently as possible," he pointed out.
Looking Towards the Future
According to the expert, today, expanding from local to city-level optimization is a costly affair, can be nearly 1000 times more expensive in fact. This assumes a tenfold increase in the area covered, which significantly complicates the optimization process. However, with advancing technology and growing demand, there are significant optimizations on the horizon. "The integration of self-driving vehicles is not far off. Some companies in the US are already experimenting with side-walk robots and self-driving vehicles. Imagine a fleet of autonomous delivery vans operating within a city, all coordinated through machine learning algorithms to sidestep traffic jams and pinpoint the most efficient routes. The potential cost savings could be enormous, but the technological and infrastructural challenges are equally significant," he said.
As Dr. Dodda aptly puts it, "The fusion of machine learning with last-mile delivery has opened doors to efficiency and convenience that were previously unimaginable. From real-time routing to the potential integration of self-driving vehicles, we're at the forefront of a revolution in how goods reach consumers. The journey has just begun, and the road ahead is filled with exciting possibilities.